Gpu Matrix Multiplication Benchmark
In order to provide a benchmark for the GPU test this study also conducted CPUbased matrix multiplication performance tests based on C language and Numpy which is a high level API widely used in data analysis. Matrix Multiplication Background User Guide.
The Best Gpus For Deep Learning In 2020 An In Depth Analysis Deep Learning Best Gpu Learning
We present performance results for dense linear algebra using recent NVIDIA GPUs.
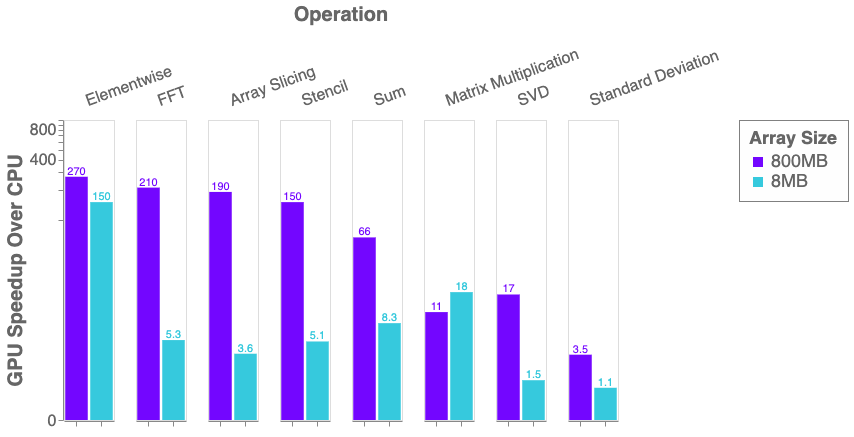
Gpu matrix multiplication benchmark. This study implements matrix multiplication based on CUDA and Tensorflow and performance test analysis. Performance Results We benchmarked our GPU algorithms and the CPU based matrix-matrix multiplication routine sgemm provided by ATLAS. This section considers the GPU implementation of one fun-damental Level 2 BLAS operation namely the matrix-vector multiplication routine for general dense matrices GEMV.
Depending on what I set BLOCK_SIZE the results become unpredictable. Tegra K1 compute capability 32 and a comparison with cuBLAS. Matrix multiplication algorithm that exploits this.
I changed everything to incorporate floats and now there is a problem. In the programming guide I coded in the matrix multiplication without shared memory access for integers and it worked perfectly. It spends around 15 of the time copying data in and out of GPU.
We tested our GPU algorithms on the ATI Radeon 9800XT. Provide LAPACK type of matrix multiplication for CUDA. This is the lowest level a wrapper of CUDA for Python.
For example Im. Similarly to sparse matrix-dense vector multiplication SpMV a desire to achieve good performance on SpMM has inspired innovation in matrix storage formatting 1618. Assuming a Tesla V100 GPU and Tensor Core operations on FP16 inputs with FP32 accumulation the FLOPSB ratio is.
For the later one we also see a breakdown of communication time between CPU and GPU. Im trying to show my boss how the GPU improves matrix multiplication by a great amount. Our LU QR and Cholesky factorizations achieve up to 8090 of the peak GEMM rate.
Cient implementations are still crucial for the performance. Tools for doing linear algebra on GPU. Implementations of a simple matrix multiplication algorithm and we compare the overall execution time.
These custom formats and encodings take advantage of the matrix structure and underlying machine. The GEMV multiplication routine performs one of. As described in the Understanding Performance section in the GPU Performance Background User Guide.
The implementation of the. This program performs matrix multiplication on various sized square matrices using the standard approach. Experiments were performed on a 3 GHz Pentium 4 CPU 512 KB L2 cache featuring peak performance of 12 GFLOPS and an L1 cache bandwidth of 447GBsec.
Our matrix-matrix multiply routine GEMM runs up to 60 faster than the vendors implementa-tion and approaches the peak of hardware capabilities. Cuda Matrtix Multiplication Benchmark. Let me first present some benchmarking results which I did on a Jetson TK1 GPU.
CPUGPU dgemm CUBLAS CBLAS Each Matrix size 12288 12288 1428 GFLOPS sustain for double precision by diving the Matrix B equally between the CPU GPU I am considering total doble precision peak for CPUGPU is 80 78 158 GFLOPS. I implemented a kernel for matrix-vector multiplication in CUDA C following the CUDA C Programming Guide using shared memory. Subscribe for more content httpsbitly2Lf16p1This video demonstrates Matrix Multiplication using Apples Metal Performance Shaders framework.
Our parallel LU running on two GPUs. Further speed up the execution we introduce the GPUs fast shared memory and. It does not use other more efficient algorithms such as the Strassen algorithm or the Coppersmith-Winograd.
Here I guess cuBLAS does some magic since it seems that its execution is. A wrapper over pycuda.
A New Approach In The Hypre Library Brings Performant Gpu Based Algebraic Multigrid To Exascale Supercomputers And The General Hpc Community Exascale Computing Project
Measuring Gpu Performance Loren On The Art Of Matlab Matlab Simulink
Pin By Ravindra Lokhande On Technical Binary Operation Deep Learning Matrix Multiplication
Python Performance And Gpus A Status Update For Using Gpu By Matthew Rocklin Towards Data Science
Matrix Vector Multiplication In Cuda Benchmarking Performance Stack Overflow
Dgemm Performance On The Cpu 4 Cores On The Gpu Including I O Time Download Scientific Diagram
Block Sparse Gpu Kernels Sparse Geometric Artificial Neural Network
Multiplication And Addition Performances On Cpu Gpu Download Scientific Diagram
Pdf The Gpu On The Matrix Matrix Multiply Performance Study And Contributions Semantic Scholar
Pdf Comparing Cpu And Gpu Implementations Of A Simple Matrix Multiplication Algorithm Semantic Scholar
Figure 7 Cooperative Cpu Gpu And Fpga Heterogeneous Execution With Enginecl Springerlink
Gpu Programming Made Easy With Openmp On Ibm Power Ibm Developer
Gpu Floating Point Operations Per Second Next We Investigate Figure 3 Download Scientific Diagram
Pin On Big Data Path News Updates
Matrix Vector Multiplication In Cuda Benchmarking Performance Stack Overflow
Speedup For Matrix Multiplication Intel 16 Core Cpu And Nvidia 448 Download Scientific Diagram